In the age of Big Data and advanced analytics, the role of the CFO has evolved to become a strategic partner in shaping business outcomes.
Master data—the core data that defines products, customers, suppliers, and other categories of business-critical information—serves as the backbone of this transformation.
Companies like Amazon and Google have demonstrated the immense value of well-managed master data, leveraging it to optimise everything from customer experiences to supply chain efficiencies.
However, the journey to clean, reliable master data is fraught with challenges, including data inconsistencies, duplicates, and missing values. These issues can lead to costly errors, such as inaccurate financial reporting and missed business opportunities.
For instance, a 2019 Gartner report estimated that poor data quality costs businesses an average of $15 million per year.
This blueprint aims to guide you through the labyrinthine process of cleaning your master data. It offers a structured approach, complete with actionable insights and practical tools, to help you not only improve data quality but also establish a robust data governance framework.
By adhering to these guidelines, you can unlock the full potential of your data, thereby driving more accurate financial forecasts, streamlining operations, and gaining a competitive edge.
Data Assessment
Master data is the lifeblood of any organisation, serving as the cornerstone for decision-making and strategic planning. However, the sheer volume and complexity of this data can make it challenging to manage effectively.
In this section, we delve into the initial steps of assessing your master data, focusing on identifying its sources, determining key data elements, and defining quality criteria.
By conducting a thorough data assessment, you lay the foundation for a more streamlined and accurate data management process.
Identify the Sources of Master Data
Understanding the origins of your master data is the first step in establishing a robust data management framework.
- Why it matters: Knowing where your master data originates is crucial for understanding its flow through your organisation. This knowledge enables you to pinpoint areas where data quality may be compromised, thereby allowing for targeted interventions.
- How to execute: Conduct an inventory of all data sources, including databases, spreadsheets, and third-party platforms. Create a data map to visualise the flow of information and identify potential bottlenecks or inconsistencies.
- Tools/Resources: Utilise tools like Microsoft Power BI or Tableau for data mapping and visualisation.
Determine the Key Data Elements Required for Analysis and Reporting
Determining the key data elements is like setting the compass for your data management journey, guiding you towards what truly matters for your organisation’s success.
- Why it matters: Identifying the key data elements is akin to setting the coordinates for a journey; it helps you focus on what’s essential for achieving your business objectives. These elements serve as the building blocks for your financial reports, analytics, and strategic decisions.
- How to execute: Collaborate with various departments to identify the data elements that are crucial for their operations and reporting. Prioritise these elements based on their impact on financial outcomes and regulatory compliance.
- Tools/Resources: Use data cataloguing software like Alation or Collibra to keep track of key data elements and their metadata.
Define Data Quality Criteria and Standards
Defining data quality criteria and standards is the linchpin that ensures your master data is not just abundant but also accurate and reliable.
- Why it matters: Without a clear set of quality criteria, your master data can become a breeding ground for errors and inconsistencies. Setting standards is akin to establishing the rules of the game; it ensures everyone is on the same page about what constitutes ‘good’ data.
- How to execute: Collaborate with stakeholders to define criteria such as accuracy, completeness, timeliness, and consistency. Document these standards and ensure they are communicated across the organisation.
- Tools/Resources: Consider using Data Quality Management (DQM) software like Talend or Informatica to automate the process of monitoring and maintaining data quality.
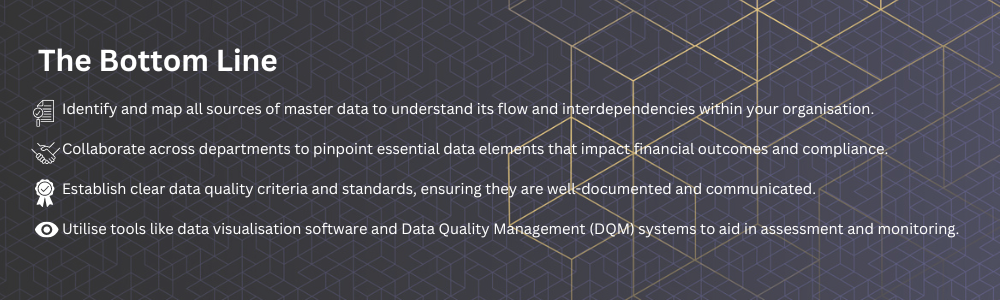
Data Profiling & Analysis
Data profiling and analysis are the investigative arms of your data management strategy. They help you dig deep into your master data, uncovering issues like inconsistencies, duplicates, and missing values that could otherwise go unnoticed.
In this section, we explore how to conduct a comprehensive data profiling exercise and analyse the impact of poor data quality on your business processes and decisions.
Conduct a Comprehensive Data Profiling Exercise
Conducting a comprehensive data profiling exercise is akin to a medical check-up for your master data, diagnosing issues before they become critical problems.
- Why it matters: Data profiling allows you to identify anomalies and inconsistencies that could compromise data quality.
- How to execute: Use data profiling tools to scan your master data for issues like duplicates, missing values, and inconsistencies.
- Tools/Resources: Tools like Trifacta and DataCleaner are excellent for data profiling tasks.
Analyse Data Patterns, Inconsistencies, Duplicates, and Missing Values
Analysing data patterns and inconsistencies is like piecing together a puzzle; it helps you see the bigger picture of your data landscape.
- Why it matters: Understanding these patterns enables you to make informed decisions and optimise business processes.
- How to execute: Use analytics tools to study data patterns and identify areas that require attention.
- Tools/Resources: Analytics platforms like Google Analytics or Adobe Analytics can be useful for this purpose.
Determine the Impact of Poor Data Quality on Business Processes and Decisions
Determining the impact of poor data quality is the reality check that quantifies the cost of inaction, making it an imperative step in your data management journey.
- Why it matters: Poor data quality can lead to incorrect financial reporting, compliance issues, and missed opportunities.
- How to execute: Conduct a cost-benefit analysis to quantify the impact of data quality issues on your business.
- Tools/Resources: Use Business Intelligence tools like QlikView or SAP BusinessObjects for this analysis.
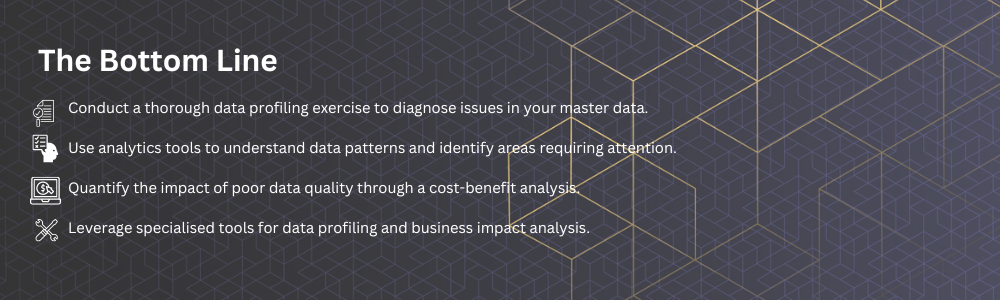
Data Cleansing
Data cleansing is the surgical procedure of your data management strategy, meticulously removing errors, duplicates, and outdated records from your master data.
This process not only enhances the quality of your data but also improves the efficiency of your business operations. In this section, we’ll guide you through developing a data cleansing strategy, implementing tools and technologies, and executing the actual cleansing process.
Develop a Data Cleansing Strategy and Prioritise Data Elements for Cleaning
Developing a data cleansing strategy is like planning a military operation; you need to identify the targets (data elements) and decide the sequence of actions to achieve maximum impact with minimal collateral damage.
- Why it matters: A well-planned strategy ensures that you focus on the most critical data elements first, optimising your resources and time.
- How to execute: Work with stakeholders to identify which data elements are most crucial and need immediate attention. Prioritise these based on their impact on business processes and compliance requirements.
- Tools/Resources: Project management software like Asana or Jira can help you keep track of tasks and deadlines.
Implement Data Cleansing Tools and Technologies
Implementing data cleansing tools and technologies is akin to equipping your troops with the right weapons; the effectiveness of your cleansing process depends on the tools you choose.
- Why it matters: The right tools can automate the cleansing process, making it more efficient and less prone to human error.
- How to execute: Evaluate and select data cleansing tools that fit your needs. Look for features like data validation, deduplication, and standardisation.
- Tools/Resources: Data cleansing software like OpenRefine or IBM InfoSphere QualityStage are good options.
Remove Duplicates, Invalid Entries, and Outdated Records
Removing duplicates, invalid entries, and outdated records is the execution phase of your data cleansing strategy, where you actively purge the system to make way for clean, reliable master data.
- Why it matters: These issues can distort your analytics, lead to incorrect reporting, and ultimately affect your business decisions.
- How to execute: Use the selected data cleansing tools to scan and remove identified issues. Always backup your data before performing any deletions.
- Tools/Resources: Backup solutions like Veeam or Acronis can ensure data safety during the cleansing process.
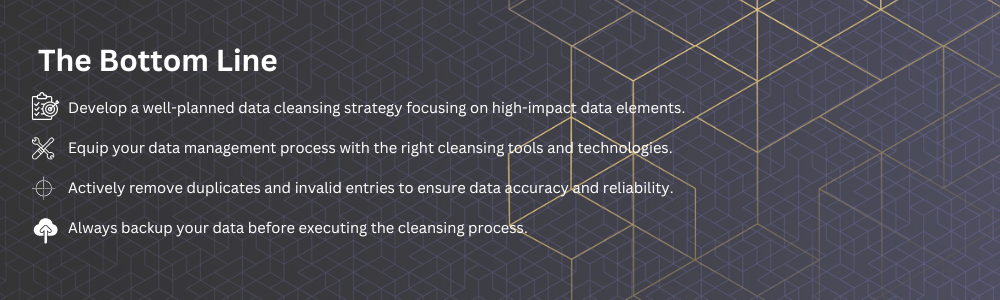
Data Enrichment
Data enrichment is the nutritional supplement of your data management strategy, adding missing elements and enhancing existing data to make it more useful and actionable.
In this section, we’ll explore how to identify missing data elements, standardise data formats, and enhance your master data with additional attributes.
Identify Missing Data Elements and Gather Additional Information
Identifying missing data elements and gathering additional information is like filling in the gaps in a jigsaw puzzle; it completes the picture and adds depth to your analysis.
- Why it matters: Missing or incomplete data can lead to skewed analytics and poor decision-making.
- How to execute: Review your master data to identify any missing elements and collaborate with departments to fill these gaps.
- Tools/Resources: Data integration platforms like Talend or Microsoft Azure can help in gathering and integrating additional data.
Standardise and Normalise Data Formats
Standardising and normalising data formats is akin to translating multiple languages into one; it ensures that everyone in the organisation is speaking the same data language.
- Why it matters: Inconsistent data formats can lead to errors and inefficiencies.
- How to execute: Implement data standardisation protocols to ensure uniformity in data formats.
- Tools/Resources: Data transformation tools like Apache NiFi or CloverDX can be useful for this purpose.
Enhance Master Data with Relevant Attributes
Enhancing your master data with relevant attributes is like adding seasoning to a dish; it brings out the flavour and makes the data more valuable for analytics and decision-making.
- Why it matters: Enhanced data provides a richer context for analytics and reporting.
- How to execute: Add attributes such as demographic or transactional data to your master data.
- Tools/Resources: Data enrichment services like Clearbit or InsideView can provide additional data attributes.
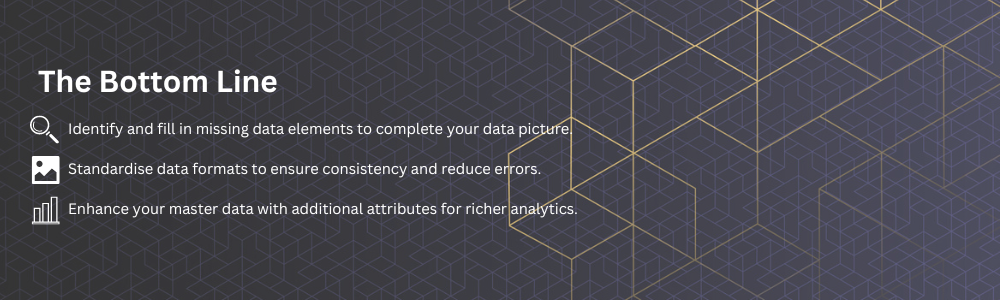
Data Validation & Verification
Data validation and verification are the quality control mechanisms of your data management strategy.
They ensure that your master data is not just clean but also accurate and reliable. In this section, we’ll discuss how to validate data accuracy against external sources, implement data validation rules, and verify data integrity.
Validate Data Accuracy Against Reliable External Sources
Validating data accuracy against reliable external sources is like cross-referencing your facts; it ensures that your data stands up to scrutiny and is trustworthy.
- Why it matters: Inaccurate data can lead to poor business decisions and compliance risks.
- How to execute: Cross-reference your data with reliable external databases or industry benchmarks.
- Tools/Resources: Data validation services like Melissa or Loqate can help in this regard.
Implement Data Validation Rules and Algorithms
Implementing data validation rules and algorithms is akin to setting up a security system; it acts as a first line of defense against data quality issues.
- Why it matters: Validation rules prevent the entry of incorrect or inconsistent data into your systems.
- How to execute: Develop and implement validation rules to check data at the point of entry.
- Tools/Resources: Data quality frameworks like Apache Griffin or Deequ can be useful for implementing validation rules.
Verify Data Integrity and Consistency Across Multiple Systems
Verifying data integrity and consistency across multiple systems is like conducting an audit; it ensures that your data remains reliable and consistent, irrespective of where it resides.
- Why it matters: Inconsistent data across systems can lead to operational inefficiencies and errors.
- How to execute: Regularly check data across different systems to ensure it remains consistent.
- Tools/Resources: Data integration platforms like MuleSoft or WSO2 can help in maintaining data consistency.
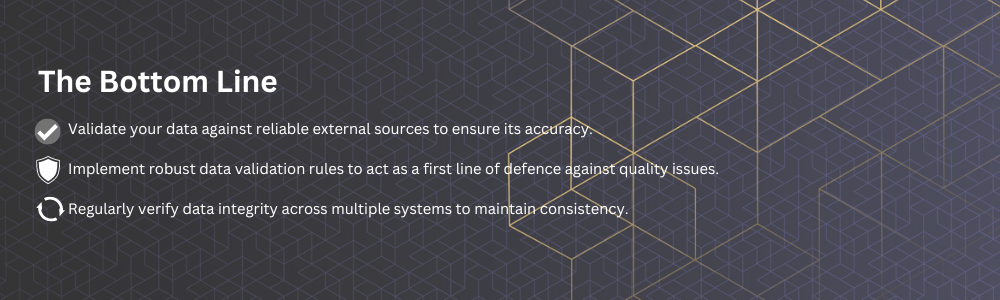
Data Governance & Stewardship
Data governance and stewardship are the legislative and executive branches of your data management strategy, respectively. They establish the rules and ensure that those rules are followed.
In this section, we’ll discuss how to establish data governance policies, assign data stewards, and implement monitoring mechanisms.
Establish Data Governance Policies, Roles, and Responsibilities
Establishing data governance policies, roles, and responsibilities is like drafting the constitution for your data kingdom; it sets the laws that govern how data is managed and used.
- Why it matters: Without governance, your data can become chaotic and unmanageable.
- How to execute: Develop a data governance framework that outlines policies, roles, and responsibilities.
- Tools/Resources: Governance software like Collibra or Informatica Axon can help in setting up a governance framework.
Assign Data Stewards to Monitor and Maintain Data Quality
Assigning data stewards is akin to appointing ministers in a government; they are responsible for specific domains and ensure that the laws (policies) are implemented effectively.
- Why it matters: Data stewards act as the custodians of data quality, ensuring that governance policies are adhered to.
- How to execute: Identify and train individuals to take on the role of data stewards in different departments.
- Tools/Resources: Training programs like DAMA CDMP or eLearningCurve can help prepare data stewards.
Implement Data Quality Monitoring Mechanisms and Periodic Audits
Implementing data quality monitoring mechanisms and periodic audits is like setting up a surveillance system; it keeps a constant eye on your data, ensuring it meets the set standards.
- Why it matters: Continuous monitoring helps in early detection of data quality issues, allowing for timely intervention.
- How to execute: Set up automated monitoring systems to regularly check data quality.
- Tools/Resources: Monitoring tools like Dataddo or Zabbix can be useful for this purpose.
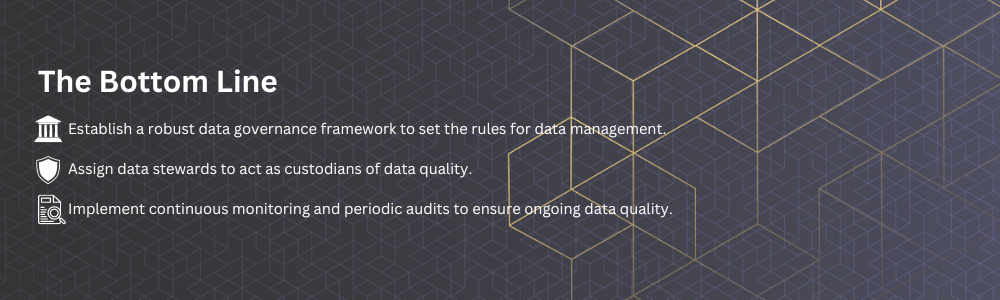
Continuous Improvement
Continuous improvement is the evolutionary mechanism of your data management strategy, ensuring that it adapts and grows with the changing needs of your organisation.
In this section, we’ll look at how to establish ongoing data quality improvement initiatives, review and refine data cleansing processes, and assess and enhance data quality practices for long-term effectiveness.
Establish Ongoing Data Quality Improvement Initiatives
Establishing ongoing data quality improvement initiatives is like committing to lifelong learning; it ensures that your data management practices continue to evolve and adapt.
- Why it matters: The data landscape is constantly changing, and your data management practices need to keep pace.
- How to execute: Set up regular reviews and updates to your data management practices.
- Tools/Resources: Quality improvement frameworks like Six Sigma or Lean can be adapted for data management.
Review and Refine Data Cleansing Processes Based on Feedback and Results
Reviewing and refining your data cleansing processes based on feedback and results is akin to iterative software development; it allows you to make continuous improvements based on real-world performance.
- Why it matters: Continuous refinement ensures that your data cleansing processes remain effective.
- How to execute: Collect feedback from users and stakeholders and use it to refine your processes.
- Tools/Resources: Feedback collection tools like SurveyMonkey or Qualtrics can be useful for gathering insights.
Regularly Assess and Enhance Data Quality Practices
Regularly assessing and enhancing your data quality practices is like going for regular health check-ups; it ensures that your data management system remains in optimal condition.
- Why it matters: Regular assessments help in identifying new challenges and opportunities in data management.
- How to execute: Conduct periodic audits and assessments to identify areas for improvement.
- Tools/Resources: Data quality assessment tools like Ataccama or Experian can be useful for this purpose.
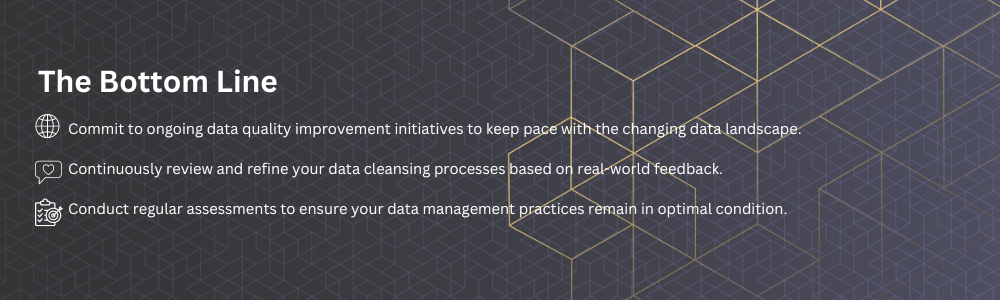
In the modern business landscape, the role of the CFO extends far beyond financial oversight. CFOs are increasingly becoming the stewards of data within their organisations, responsible for ensuring that master data—the backbone of any data-driven strategy—is clean, reliable, and actionable.
This blueprint has been designed as a comprehensive guide specifically for CFOs who are looking to take charge of their master data management initiatives.
From the initial steps of data assessment to the ongoing commitment to data quality improvement, each section of this blueprint has been crafted to provide CFOs with actionable insights, practical tools, and a structured approach.
By following this guide, CFOs can lead their organisations in establishing a robust data governance framework, thereby achieving data excellence. This is not just about cleaning data; it’s about leveraging clean, high-quality data to make more informed decisions, drive operational efficiencies, and gain a competitive edge in the market.
The role of the CFO in master data management is pivotal. You are the linchpin that connects data with strategy, analytics with action, and insights with outcomes.
By taking a proactive role in cleaning and managing master data, you are not just enhancing data quality but also building a data-centric culture that supports long-term business objectives and growth.
Was this article helpful?
YesNo